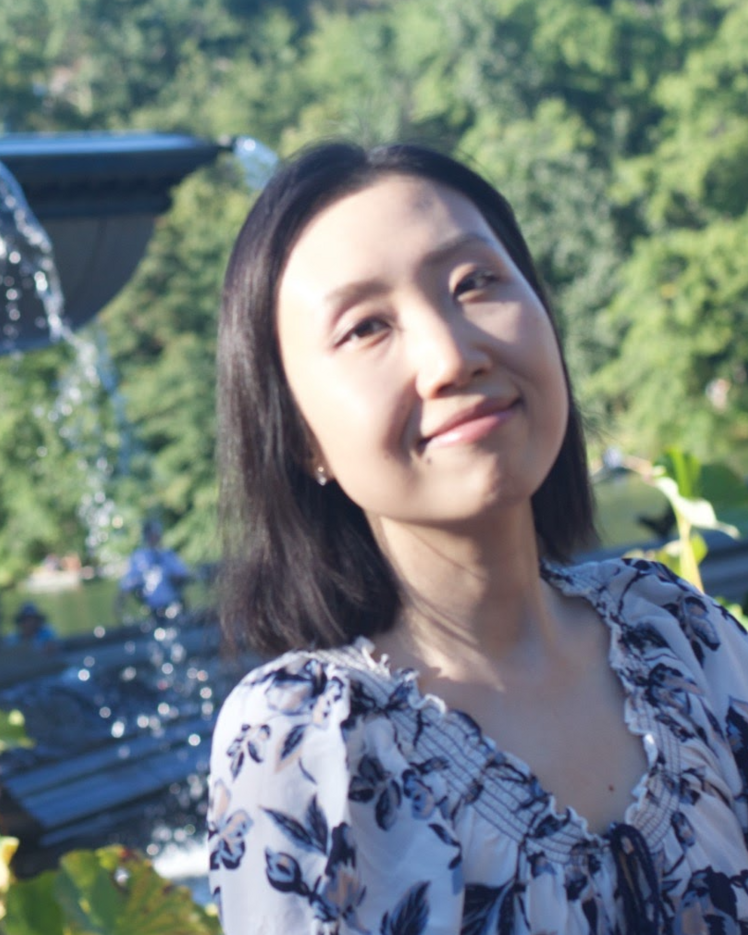
Jin Guo
Biography
Jin L.C. Guo is an assistant professor at the School of Computer Science, McGill University.
She is interested in using AI techniques to solve software engineering problems. Her recent research focuses on mining domain knowledge from software traceability data and using such knowledge to facilitate automated SE tasks, such as trace retrieval and project Q&A.
Guo completed her PhD at the University of Notre Dame. Prior to that, she worked on image processing and computer vision in Fuji Xerox’s research lab.